Many organizations claim that data is an important asset to them. But at a closer look data is still a by-product for most of them and frustration about failing data initiatives is wide spread. This especially applies to organizations which assess data and their use as a competitive advantage.
Our experience: It’s (often) not about technology. Organizations need a mindset shift when it comes to data. Treating Data as a Product helps organizations to effectively manage and utilize the data they collect and produce.
A Shifting Mindset: (Data) Customer is King!
One of the key benefits of Data as Product in the context of Data Mesh is that it allows organizations to take a more customer-centric approach to data. By treating data as a product, teams are forced to think about the value that their data provides to different stakeholders, and how to best deliver that value. When successfully implemented, it changes organization’s view on data handling.
This can lead to more focused and effective data management, as well as greater alignment between data teams and business units. By treating data as a product, organizations can foster innovation and experimentation. This unlocks the full value of their data assets.
What does “Data as a Product” mean?
At its core, data as a product refers to the idea that data can be treated as a standalone product, with its own value proposition, revenue stream, and lifecycle management.
Within the so-called data management concept Data Mesh the principle “Data as a Product” applies product thinking to data. It comes down to two main interpretations:
- Data is treated as a product
- Therefore, consumers of data (e.g., other teams) should be treated as customers
Within data projects data preparation (e.g., data loading, data cleansing) often is one of the major time-consuming tasks. Projects can fail due to missing accessibility or quality. Data as a Product changes the perception of data within an organization and therefore directly addresses these challenges. Data is not a by-product anymore.
The founder of data mesh Zhamak Deghani defines 8 attributes to describe the usability of data product: Discoverable, addressable, understandable, trustworthy, accessible, interoperable, valuable and secure.
To be part of the Data Mesh these baseline attributes need to be fulfilled. As an example: Domain teams should be enabled to explore and search organization-wide data sets for their data use cases. This can be realized by providing a central data catalog (discoverable). The data sets presented should be permanently addressable using an organization-wide convention (addressable). Data Security refers to access controls, confidentiality (e.g., personal identifiable information), GDPR and other specific regulations that can apply to a business (secure).
What is the difference between Data Products and Data as a Product?
The terms Data as a Product and Data Product are sometimes used interchangeably but there is a difference. Data as a Product is part of the strategy, while Data Product is the outcome of that strategy.
As described above Data as a Product is one of the four builiding blocks of the so-called data management concept Data Mesh. Data as a Product applies product thinking to datasets and therefore represents a set of beliefs. The principle is sometimes shortened to Data Product.
Data Product refers to the actual product that is created from data. It is a tangible or intangible product that is the result of the process of turning data into something that can be sold to internal or external customers. Data Product can be a report, dashboard, API, dataset, algorithm, software, service, etc.
An Example for Data Products
The CRM team includes individual product recommendations based on transactional data, user’s web and app behavioural data into their mailings. This feature is a data product since data is the key enabler for its primary goal. Within the understanding of Data as a Product this data product example can be structured as follows:
- Data product consumer domain is the CRM domain
- Data product consumers is the product affinity mailing and after sales mailing
- Data product job is to enable personalized and relevant content
- Data product producers (sources) are analytics (for user-related web and app behavioral data) and transasctional data
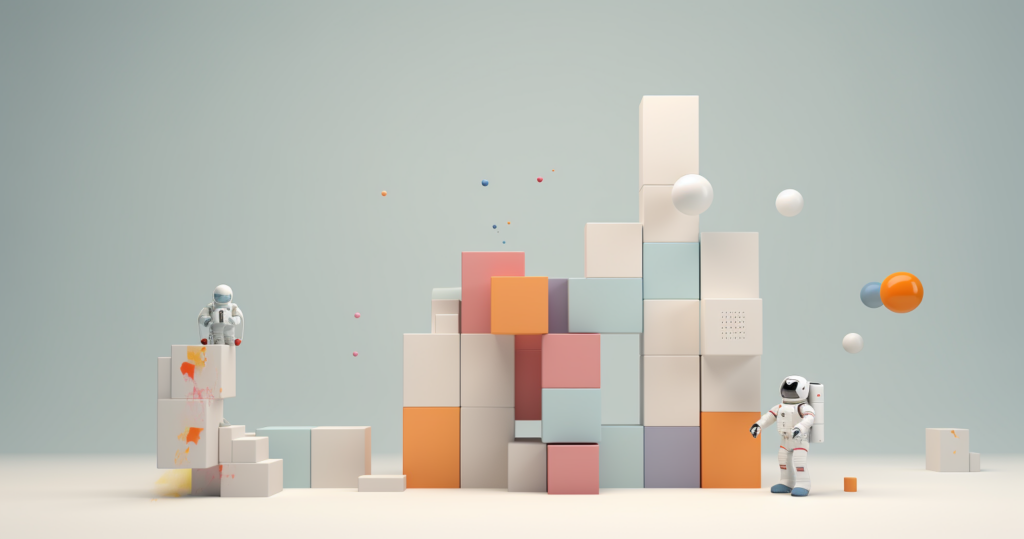