Data mesh is a sociotechnical concept for a decentral data architecture. The paradigm is a shift in data management that addresses the challenges of large organizations that often struggle to deliver business value and impact from data. The basis for data mesh are four principles:
- Data as a product
- Domain ownership
- Self-serve data infrastructure
- Federated computational governance.
A mesh is a network of access points or nodes that are linked together.
Most organizations rely on central data teams with a central data lake or warehouse. The expectation is that they drive business with their data products. In the beginning this often works but they quickly become a bottleneck and the question of priorities and waiting-in-line for business teams begins. These and other challenges for data management are the starting point for data mesh. Data mesh is an answer to the limitations of earlier data management paradigms such as data warehousing and data lake. The final concept of data mesh has been developed and promoted by Zhamak Dehghani in her role as director of emerging technologies at Thoughtworks.
What is Data as a product?
One of the fundamental building blocks of data mesh is Data as a Product. It refers to the way data is treated within an organization. Within this paradigm data sets are seen as products and the people within the organization (e.g., data engineers, data scientists) become customers. Domain teams provide their data set to the rest of the organization who can consume it for their data products. The principle applies product thinking to data sets. This means in the end: data is treated like a product, not like a by-product.
To make data sets consumable a set of capabilities is needed such as discoverability, addressability, understandability, self-description, security, trustworthiness and interoperationality. As an example: A “customer” finds data sets via internal search engines. The data sets are self-describing and include the location. Moreover, sample data and exemplary SQL queries using the data set are provided.
What is domain ownership?
Data mesh addresses this challenge by introducing domain-oriented decentralized ownership. Domain teams consist of people typically organized around a common business purpose. Examples of domains could be marketing, product, checkout, return, etc. Domain teams know their data best and they are responsible for providing their data to other domain teams. It’s the responsibility of the domains to make their data accessible, usable and to ensure that it meets the standards set by the federated governance. Domains are owners of data products but also producers of data products. This principle represents a shift of data ownership from centralisation to decentralisation.
What is a self-serve data infrastructure?
Central data teams are often a bottleneck in the way of efficient data usage. This can lead to frustration, increasing development time or even failure of data projects. Domain teams are enabled to work, develop, and maintain their data product independently. They get quicker access to the data and therefore speed up time to market. It better supports scalability than central approaches where resources are often scarce.
What is a federated computational governance?
The missing piece to complete data mesh is federated computational governance. Decentral ownership and self-service lead to questions of rules and ways of working. The principle federated computational governance aims at providing interoperability of all data products and collaboration through standardization and guidelines. This allows for the combination of data products from different domains, security, and compliance. Standardization can include documentation standards, organizational governance policy standards, and industry regulations.
Why is the concept of data mesh so popular?
The socioeconomic concept of data mesh becomes more and more popular because especially large organizations have a high failure rate for data projects. Organizations see the use of data as an important strategic asset but there is frustration about the realization. Over the last years few ideas but data mesh has gained so much attention and support in data management practice.
As an example: A study of VentureBeat (2019) showed that 87% of data science projects never make it to production. Here is a glimpse of the reasons: One reason is scattered data, meaning data is lying in silos across various teams and the coordination of these teams across the organization is inefficient. With its four principles data mesh addresses these challenges and therefore offers a fundamentally different answer to data management in an organization. Companies like Zalando, adidas, Delivery Hero and HelloFresh use data mesh for their data management.
#applydatamesh as a resilient foundation for data management
At diconium data we believe that large organizations aiming at driving business through data need to create a data culture in which ownership, accountability and ability to progress is given back to decentral teams. Data mesh paradigm provides the answer to a number of today’s data management challenges and offers a resilient foundation for getting value from analytical data at scale. We call this #applydatamesh.
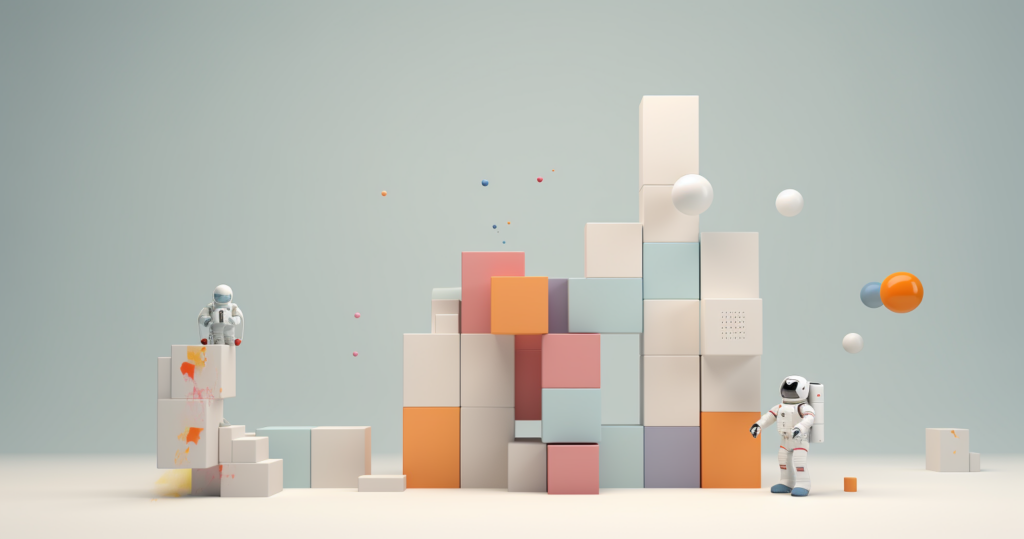