Data management is an organizationās practice of collecting, storing, processing and securing its data, with the objective of maximising the value of using data for all the different business processes. Find out how to future-proof your business by leveraging the most prominent data management trends, brought to you by diconium data.
In this article, we are reviewing 5 of the most disruptive trends we have identified in our practice driving digital transformation in organizations.
1. Automation and AI
To address the explosion of data, more and more enterprises are embracing generative AI to augment their capacity to derive value from it. Artificial intelligence solutions are shaping the future of business intelligence by enhancing human insight and execution.Ā
One popular use case is sentiment analysis, a technique which uses a mix of natural language processing, linguistics, text analysis, and algorithmics in order to infer the emotional charge of user-generated text over the internet. Social media monitoring tools have jumped at the opportunity to build products which mine emotions among online communities and empower informed messaging strategies.
Building own applications
The beauty of living in the digital age, however, is that organizations are not confined to any vendorās walled garden. Businesses nowadays can build their own applications for internal consumption by using open-source tools, such as Google Cloud Natural Language API.
Using Googleās BigQuery, technical teams have all the necessary equipment to build a cost-efficient data product. Furthermore, they can create a dashboard in Looker Studio (formerly known as Data Studio) ā a free visualization tool from Google ā which can provide a friendly interface for other teams to consume the data.
ChatGPT in use
Another iconic use of Artificial Intelligence is none other than ChatGPT, the chatbot trained by OpenAI, which has lately been on everyone’s lips. Its use cases range from spinning out blog posts to solving coding problems. While its reception has been mostly positive and the AI model is set to revolutionize the future of work and technology, it may be a long time before artificial intelligence becomes widely implemented. Those who embrace AI and progressively integrate it into their organizations will undoubtedly reap the first moverās advantage as the technology advances.
Watch ChatGPT in action:
2. Edge Analytics
No sooner have digital organizations designed efficient data management systems for ātraditionalā data sources, than they are faced with a new challenge. The rise of connected devices and IoT systems calls for revamped strategies where real-time processing is of the essence.
Insights into our work
In our long-standing history within the mobility sector, we have tapped into edge analytics for several projects, two of which we like to think stand out as particularly bold. While conscious of our clientsā privacy (speaking of which, we might dedicate an entire article to secure data management trends), we would like to share a brief overview of how we deal with data at the edge.
Car data
Working with a German car manufacturer during the months leading up to the release of their e-car model, our colleagues developed an app which integrates seamlessly with charging stations. The solution allows car owners to access charging services data from the edge and plan their trips in advance with full predictability regarding providers, locations, connections, and more.
Monetization of data
For the second project, we partnered up with a B2B automotive company which collects tremendous amounts of sensor data points. Our mission was to gauge the financial potential of their data assets, which brings us to a critical point when discussing data capital ā monetization. Because all businesses are in the money business, edge analytics can provide valuable monetization sources for companies looking to diversify their revenue streams.
3. The Rise of DataOps
DataOps is a set of practices within a collaborative data management model, which aims to bridge the gap between domain data owners and data consumers. To ensure the delivery of value from data, organizations need to accelerate the use of analytics and to empower data-driven decision making.
Why DevOps?
Following in the footsteps of methodologies such as DevOps and DevSecOps, DataOps teams come together to accelerate the velocity of analytics in the company, translating it into actionable business intelligence. The methodology of DevOps is extended with data specialists such as data engineers, and data scientists. The goal is to enable data streams and the ongoing use of data across the enterprise.
In a 2020 report, Seagate US found that an average of 10% or surveyed companies deployed DataOps organization-wide.
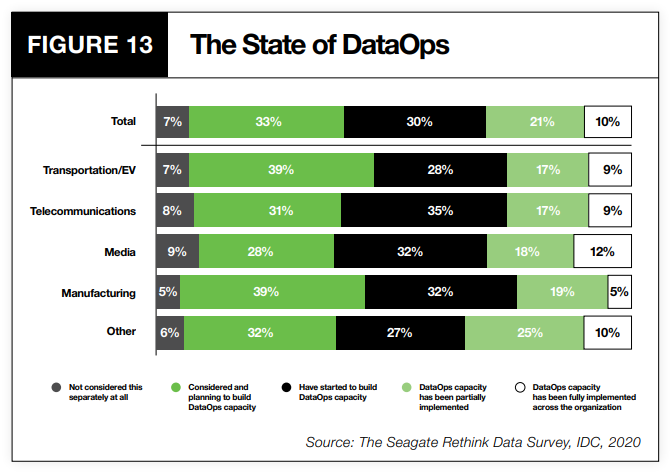
By contrast, a whopping 89% of respondents deem DataOps to play an important role in the company.
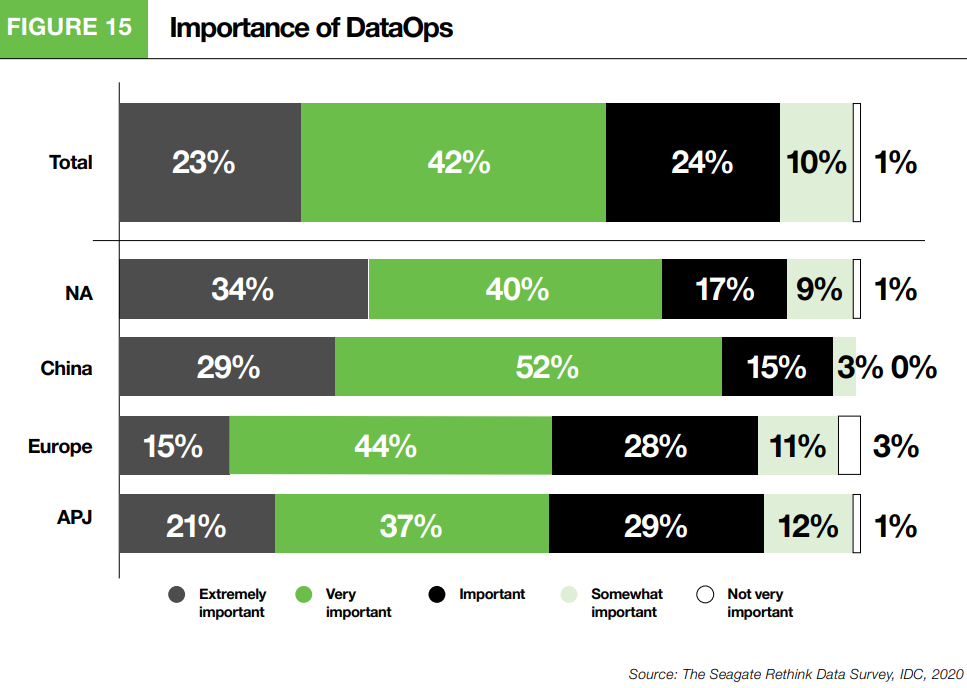
The results indicate that there is huge untapped potential for businesses to invest in DataOps teams and to enhance value delivery from their data, thus obtaining a competitive advantage.
4. Self-Service Data Infrastructure
Shifting towards a self-serve setup instead of relying on central data availability empowers teams to work independently. This translates into faster time to market and is especially relevant for large enterprises where not everybody knows who is responsible for what. Many of the roadblocks that occur in traditional organizations are thus removed, allowing for increased efficiency and self-sustainability.
One example of valuable assets that teams have access to in a self-serve infrastructure consists of catalogs of data sets. You can think of these like internal repositories of data that all the relevant teams can query and/or use for the scope of their work. Decentralized teams throughout the organization are no longer reliant on a central analytics team with limited resources.
Independence and efficiency in data product development
A self-serve data infrastructure is a prerequisite for domain teams to achieve the necessary independence to build and own their respective data products. Organizations that want to scale sustainably and to unlock the full potential of their business intelligence need to take steps towards removing bottlenecks and speeding up time-to-value for their data.
Designing inclusive data infrastructure for all skill levels
However, simply decentralizing the responsibility for data ownership and maintenance is not enough. The company-wide data infrastructure needs to accommodate various levels of expertise and tech proficiency by ensuring a shared platform and tools that non-technical business teams can easily grasp.
Data mesh principles
As a decentralized organization, we are eager to stay on top of the latest data management trends, all the while approaching them with a critical eye. That being said, we are huge fans of the principles proposed by data mesh. For anyone in the space, it should come as no surprise that self-service data infrastructure made it to our top 5 data management trends for 2023. (If you want to learn what a data mesh is, check out the article our colleague lovingly wrote for you.)
5. Data Fabric ā the Up- and Coming Data Management Trend
Siloed data has been plaguing enterprises, hindering innovation and giving more digitally-savvy competitors the upper hand. Data fabric technologies come in to address such data governance shortcomings inherent to traditional data management frameworks.
Understanding data fabric architecture
Data fabric is a term that is used to describe a data management architecture that is designed to provide a seamless and consistent way to access and manage data from various sources, including on-premises systems, cloud-based systems, and other types of data stores. A data fabric typically includes a set of tools and technologies that enable data integration, data management, and data governance across an organization’s entire data ecosystem. The goal of a data fabric is to make it easier for organizations to access and use their data in order to gain insights, make better decisions, and improve business outcomes.
According to Gartner, data fabric deployments will improve the efficiency of data usage by 400% and will reduce the need for human involvement in data management by half.
Data fabric vs. data mesh: key differences
But what is the difference between data fabric and data mesh? While both architectures emerged in order to mitigate the challenges that businesses face in terms of data management, ādata fabric is technology-centric, while a data mesh focuses on organizational changeā explains James Serra, Data & AI Solution Architect at Microsoft.
What a data fabric ultimately does is to enable higher efficiency through the use of ML/AI, all the while empowering business teams to act autonomously as data consumers and to derive actionable insights.
Automotive Extra: Self-Driving Cars
Over the course of our existence, we at diconium have built a reputation as a trusted partner for mobility and automotive clients, and built extensive expertise in cybersecurity, infotainment, and autonomous driving.
ADAS (Advanced Driver Assistance Systems) advancements are driving innovation in the self-driving car market, and increasingly qualitative datasets are paving the way for accelerated developments.
The future of autonomous vehicles
Market research firm Next Move Strategy Consulting estimated a global autonomous vehicle market of 17,000 units in 2022, and the market is projected to projected to reach the size of some 127,000 units in 2030. (Statista)
What other data management trends do you anticipate will gain momentum in the coming years? Reach out to our colleagues to discuss how your organization can #applydatamesh to enhance its value from data.