As the amount of data generated by businesses continues to grow, so does the need for advanced analytics techniques that can help organizations gain meaningful insights and make data-driven decisions. From machine learning and artificial intelligence to predictive modeling and data visualization, the field of advanced analytics is rapidly evolving, providing businesses with new ways to analyze and leverage their data for competitive advantage.
In this article, let’s explore some of the latest trends and technologies in advanced analytics, discuss how businesses can use them to unlock insights, improve operations, and drive growth and value the contribution of data analysts. In our upcoming blog series, we will explore a range of analytics toolsets, covering essential aspects like KPI definition, web and app analytics, and marketing analytics, enabling readers to unlock the power of data-driven decision-making. Additionally, we will delve into advanced analytics techniques, including user segmentation, retention analysis, A/B testing, and predictive analysis, providing insights into common methods for driving valuable insights from data.
Defining KPIs carefully
The first step of conducting a successful data analysis after collecting the necessary data is defining Key Performance Indicators (KPIs). They are used to evaluate the success of an organization or a particular activity and are commonly used in every business to measure overall performance. They are insight-driven and differentiate from goals, and are aligned to short or long-term business objectives. KPIs serve as a benchmark for day-to-day activities, are flexible, and should be “SMART” – specific, measurable, attainable, realistic, and timely. To create effective KPIs, it’s important to track and report them appropriately and accurately, and they should be understood by everyone within the organization. We have previously published a post on constructing well-designed dashboards for KPIs.
Here is thefull article about how to define KPIs for impactful insights.
Analytics for websites vs. mobile applications
App analytics and web analytics both involve tracking user behavior to gain data-driven insights, but they differ in terms of platforms, tracking units, and metrics. Web analytics measures the performance of a website, while app analytics is for mobile apps. In web analytics, metrics such as pageviews, unique visitors, bounce rate, time on site, conversion rate, traffic sources, exit pages, and landing pages are important, depending on the website’s goals and objectives. In contrast, product analytics for mobile apps track metrics such as activation rate, feature usage, and retention rate, to determine which aspects of the product are most valuable to users or represent key steps in their journey. Product analytics key metrics can be organized into four categories: acquisition, engagement, retention, and monetization. By analyzing these metrics, product teams can continuously improve the product by measuring, experimenting, and evaluating changes and releases.
Here is the full article Exploring mobile app analytics vs. website analytics.
Marketing analytics
Marketing analytics is a data-driven approach that measures and analyzes the effectiveness of marketing campaigns and activities. Marketing professionals use web and app analytics, social media analytics, customer analytics, and marketing automation algorithms to collect and process data. The goal is to maximize return on investment (ROI) by delivering the right message to the right audience at the right time. Marketing analytics evaluates the success of marketing efforts in driving either conversions, brand awareness, or both. By analyzing user behavior, marketing professionals can identify the most effective channels, campaigns, and messaging for reaching their target audience. Marketing analytics provides businesses with deeper insights into their target consumers, creating more targeted and effective marketing campaigns, personalized product offerings, and better customer experiences. Marketing analytics is critical for optimizing ROI by tracking and analyzing data from marketing campaigns. Marketing channels refer to the different ways businesses use to reach and engage with their target audience. Cross-channel marketing refers to the practice of using multiple marketing channels in a coordinated way. Customer lifteme value (CLV) and customer acquisition cost (CAC) are two essential metrics that businesses use to evaluate the profitability and effectiveness of their marketing efforts. Attribution models are rules or algorithms that businesses use to assign credit for conversions to different marketing channels and touchpoints along the customer journey.
Here is the full article: Marketing Analytics: Improving the Success of Marketing Campaigns.
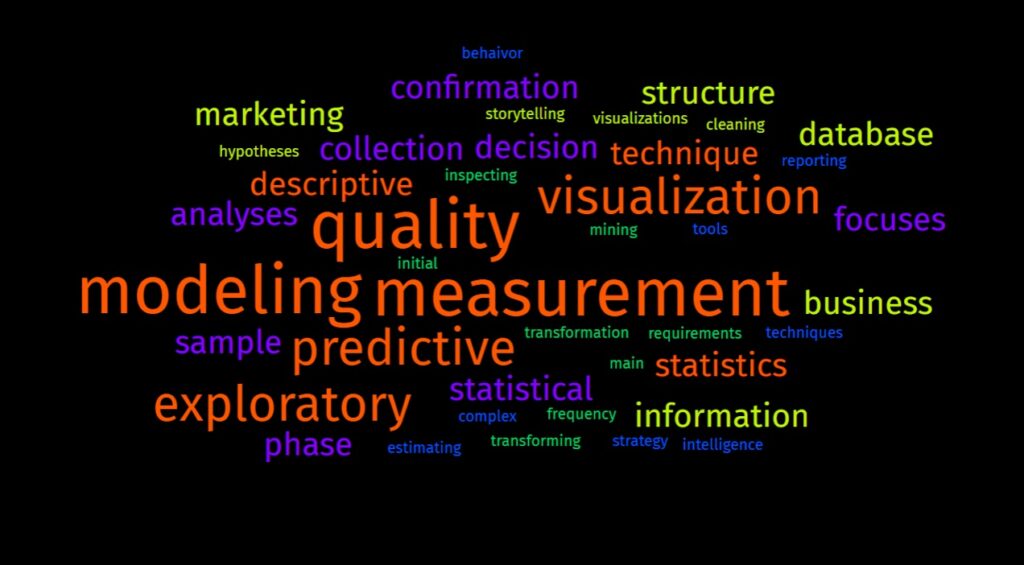
Advanced analytics methods
1. Segmentation and cohort analysis
By grouping similar data into different categories based on the chosen criteria, data segmentation allows analysts to give more effective, actionable insights for product development and marketing. To gain a deeper knowledge of the users or customers, segmentation may be used in conjunction with a variety of analysis. Basic segmentation techniques include those that are based on the demographics of the users or their geographic location, as well as more advanced and behavioral techniques like RFM (recency-frequency-monetary) segmentation, power users, revived users, or feature-based users.
2. Churn & retention analysis
Retention and churn analyses play a crucial role in product analytics by identifying the underlying factors that influence whether users continue using a product or service. These analyses offer valuable insights to optimize user retention strategies and mitigate churn risks, ultimately driving business growth. Retention rate is the percentage of users who continue to use a product or service over a given period of time, while churn rate is the percentage of users who stop using a product or service over a given period of time. By combining cohort and other segmentation methods, powerful insights can be derived on how user retentions differ based on different characteristics or feature usage, allowing teams to develop strategies for reducing churn.
Here is the full article about Product Analytics: Cohort and Retention Analyses.
3. A/B tests
A/B testing is a method that aims to measure the causal impact of a specific change, independent of other influences and factors that could affect the outcome. This is commonly used in digital marketing to optimize conversion rates and user engagement. The process involves randomly assigning subjects to either a control group or a treatment group and measuring a specific metric (such as click-through rate or conversion rate) to determine if the treatment group performs significantly better than the control group. Statistical techniques can be used to analyze the results of A/B tests, such as hypothesis testing, and regression analysis. By conducting an A/B test, businesses strive to isolate the effects of the change they are testing and determine its direct impact on user behavior or performance metrics.
4. Predictive analysis
Predictive analytics uses historical data and current information to predict future outcomes and trends, and identifying the best model for a business is crucial for success. The process involves understanding the business objective, data preparation, model training, results analysis, and model deployment. Predictive models need to be validated and retrained regularly for optimal performance. The top five predictive analytics models are classification, clustering, forecast, outliers, and time series. Predictive analytics enables businesses to anticipate future trends, customer behavior, and potential risks, and empowering them to make informed decisions. It offers valuable insights for businesses in specific areas such as optimizing marketing campaigns, forecasting demand for products or services, identifying fraud patterns, predicting equipment failures, and improving customer retention strategies.
Here is the full article about Product Analytics Part II: Predictive analysis.