In the world of data-driven decision-making, understanding user behavior and preferences is crucial for successful product development and marketing. In this post, we will delve into data segmentation and cohort analysis, shedding light on how these techniques provide actionable insights. We also explore specific segmentation methods like RFM segmentation, power user analysis, resurrected user analysis, and cohort analysis, offering a comprehensive foundation for harnessing data in the pursuit of effective strategies. Additionally, we touch on churn and retention analysis, vital tools for gauging user engagement and attrition. Join us as we embark on a journey through the essential facets of data-driven decision-making for product development!
This post is part of our Analytics Beyond Dashboards series. Please take a moment to explore our previous posts in the series:
- How to Define KPIs for impactful insights
- Mobile App Analytics vs. Website Analytics
- Marketing Analytics: Improving the success of marketing campaigns
Segmentation and Cohort Analysis
Data segmentation is dividing data by putting related ones in various categories depending on the selected criteria and it enables analysts to provide more efficient actionable insights in product development and marketing. Segmentation could be coupled with many analyses to have a better understanding of the users or customers. There are basic segmentation methods such as based on demographic features or geographic situation of the users as well as complicated and behavioral ones such as RFM segmentation, power users, resurrected users, or feature-based users.
Those segments help understand the user’s motivation to engage with the product or customer behavior towards being a user. For some products for instance, cultural behaviours could matter in terms of usage or subscription of the product. In such cases, monitoring the user behaviours by countries provides better insights to decision makers. Similarly, stickiness or level of engagement of users who organically install the product and the users who get to know the product via various marketing channels could differ. Based on the domination of either group of users, a type of new feature release could be reconsidered or redesigned.
There are more specific behavioural segmentation methods such as RFM, power users, resurrected users, cohort analysis, etc.
Let’s have a closer look at some examples of those segmentation methods.
RFM segmentation
One of the widely used ones in e-commerce is RFM segmentation. It is a technique used to segment customers based on their recent purchasing behavior. RFM stands for Recency, Frequency, and Monetary Value.
- Recency: Refers to how recently a customer has made a purchase. Customers who have made a purchase more recently are typically considered more valuable and may be targeted differently than those who have not made a purchase in a while.
- Frequency: Refers to how frequently a customer makes purchases. Customers who make purchases more frequently are typically more valuable than those who make purchases less often.
- Monetary Value: Refers to how much money a customer has spent on purchases. Customers who have spent more money are typically considered more valuable and may be targeted differently than those who have spent less.
RFM segmentation typically involves assigning scores to each of these three criteria, and then grouping customers into categories based on these scores. For example, a high-scoring customer might be considered as champion customer and receive targeted marketing and promotions, while a low-scoring customer might be targeted with re-engagement campaigns.
RFM segmentation is often used in e-commerce and retail but can be applied in other industries as well. It is a relatively simple and effective way to segment customers based on their purchasing behavior, allowing companies to tailor their marketing.
Power users
Whereas RFM segmentation is used widely in e-commerce industry, power user analysis is a solid tool to gain insights regarding the users in SaaS or similar products and services. Power user analysis involves identifying the most engaged and active users of a product, and analyzing their behavior and usage patterns to gain insights that can be used to improve the product and increase user engagement.
Metrics such as frequency of use, time spent using the product, number of features used, and engagement with the community are utilized to identify power users. Once power users have been identified, their behavior can be analyzed to identify patterns and insights that can inform product development and marketing strategies.
Here are some examples of insights that can be gained from power user analysis include:
- Identifying which product features are most popular among power users and using this information to prioritize feature development and marketing efforts.
- Analyzing power user engagement with the product community, such as participation in forums or sharing of user-generated content, to identify opportunities to improve community engagement and foster brand advocacy.
- Examining power user usage patterns over time to identify trends and opportunities for improving user retention and reducing churn.
Resurrected users
Like power user analysis, resurrected user segmentation is a valuable tool in product analytics. Resurrected user analysis is a type of product analytics that focuses on understanding and analyzing users who have returned to a product or service after a period of inactivity or churn. The aim of resurrected user analysis is to understand why these users left in the first place, why they returned, and what factors influenced their decision to come back.
To conduct a successful resurrected user analysis, some questions need to be answered with measurable metrics. For example, how long did users remain inactive before returning to the product or service? How often are resurrected users using the product or service? Which features of the product or service are most used by resurrected users? By analyzing these metrics, product teams can gain insights into the factors that influenced resurrected users to return to the product or service. This information can then be used to develop strategies for reducing churn and increasing user retention.
Cohort analysis
Cohort analysis is a type of user segmentation that is time period specific. It is again a widely used tool in product analytics especially SaaS businesses. The goal of cohort analysis is to understand how changes to the product or service affect user engagement and retention over time. Product managers can identify months and seasonal trends easily when the product performs well or poorly in terms of revenue generation, new subscriptions, customer churns, etc. by using cohort analysis.
As a type of segmentation, cohort analysis, differs from user segmentation by being time-bounded analysis. Cohort analysis focuses on understanding how changes to the product or service impact user behaviour over time, whereas segmentation analysis focuses on understanding the characteristics and behaviour of various groups of users. This is the main distinction between the two methodologies.
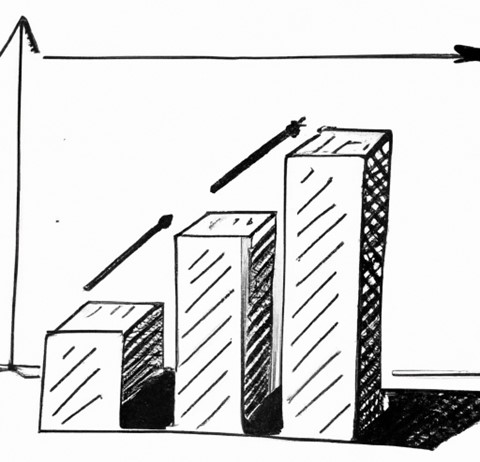
Churn & Retention Analysis
Churn or retention analyses is another significant type of analysis widely used in product analytics. Retention and churn rate are mathematically complementary.
Let’s start with retention rate definition and calculation method. Retention rate is the percentage of users who continue to use a product or service over a given period. It is calculated as the number of active users at the end of a period divided by the number of active users at the beginning of that period.
Here is the formula for calculating retention rate:
Retention rate = (number of users at the end of a period / number of users at the beginning of the period) x 100
For example, if a product has 10,000 active users at the beginning of a month and 8,000 active users at the end of the month, the retention rate for that month would be:
Retention rate = (8,000 / 10,000) x 100 = 80%
Churn rate is the percentage of users who stop using a product or service over a given period of time. It is calculated as the number of users who churned during the period divided by the total number of users at the beginning of the period.
Here is the formula for calculating churn rate:
Churn rate = (number of users who churned during the period / number of users at the beginning of the period) x 100
For the same example above,
churn rate = (2,000 / 10,000) x 100 = 20%.
While retention and churn analysis are related, they are not the same thing. The goal of retention analysis is to understand how well a product or service is retaining its users and to identify factors that contribute to user retention. The goal of churn analysis, on the other hand, is to identify the factors that contribute to user churn and to develop strategies for reducing churn.
Retention and churn analyses basically using cohorts as they are segmented based on a specific period. When those are combined with other segmentation methods, such as behavioral, geographic, or demographic, powerful insights could be derived on how user retentions differ based on different characteristics or feature usage. For example, segmenting users based on their onboarding experience can help teams identify areas where the process can be improved. If users who complete a certain tutorial or feature are more likely to stay engaged, the team can focus on improving that aspect of the onboarding experience.