“I have a dream …”
– Martin Luther King –
When I started to work at diconium in mid-October 2021, within my first main project, I had the task to research the question how one could systematically build processes for developing traceable and reproducible AI systems in the safety relevant domain of autonomous driving. We needed to research new ways to evaluate and assess AI elements within larger software systems. In what way or what type of dimensions one could and should assess these AI elements was mostly not fully clear to us at that time. It was within that project where I came up with the concept of a Continuous AI Assurance framework. The idea, in principle, of such a framework is to ensure the “quality” of an AI unit within a safety relevant software along the whole development cycle. In February 2021 I wrote a first article on the need for such a framework on the diconium applydata Blog.
I had suddenly this dream forming in my mind: What if we build an intelligent system that is enabling the continuous deployment and update of any safety-relevant software (e.g., in automotive, medical, industrial, energy, and financial domains) with AI/ML elements integrated within them, while being safe and agile at the same time. Imagine as a metaphor a futuristic large factory site with a conveyor belt prominently placed in the center of the factory (see Fig. 01 for a Midjourney imagined dream of such an AI factory). On this conveyor belt we see dozens of mysterious cubes. Imagine these cubes to stand for different AI elements that are developed in a proverbial AI factory. The imagined factory site with the conveyor belt and the assessing instruments then stands for our continuous AI Assurance system.
Playing a bit further with our metaphorical game, the aim of the Continuous AI Assurance framework would be to essentially opening these “obscure” boxes and evaluating their inner workings down to the last part within them (see Fig. 02 for a Midjourney imagined dream of such an AI element as opened black box) for the sake of evaluating the parts alone and in unison.
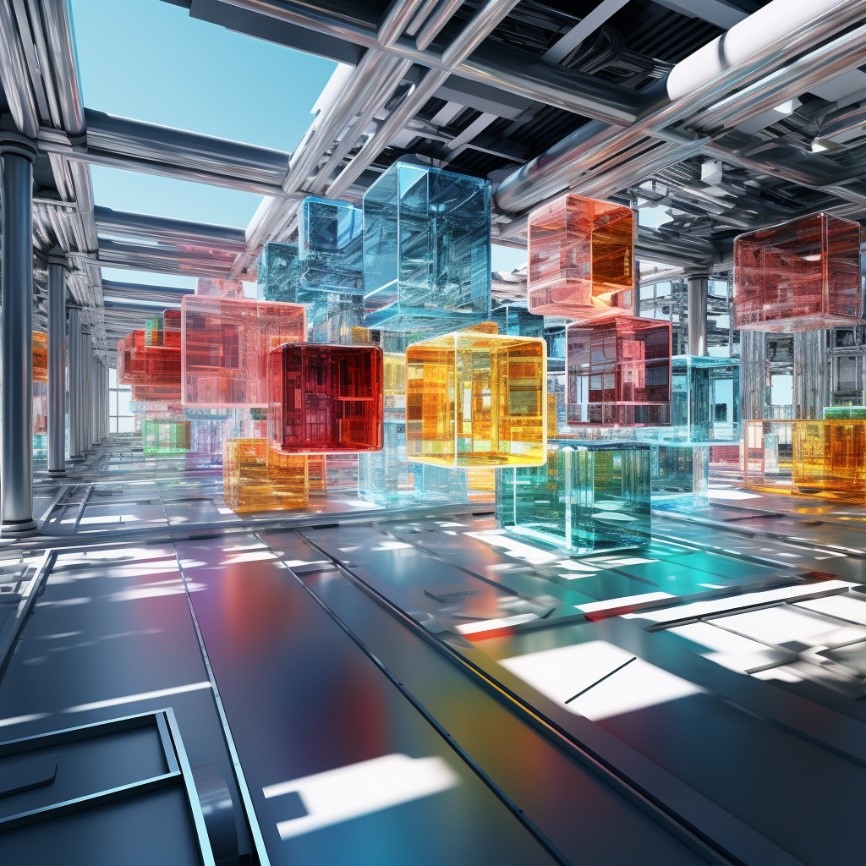
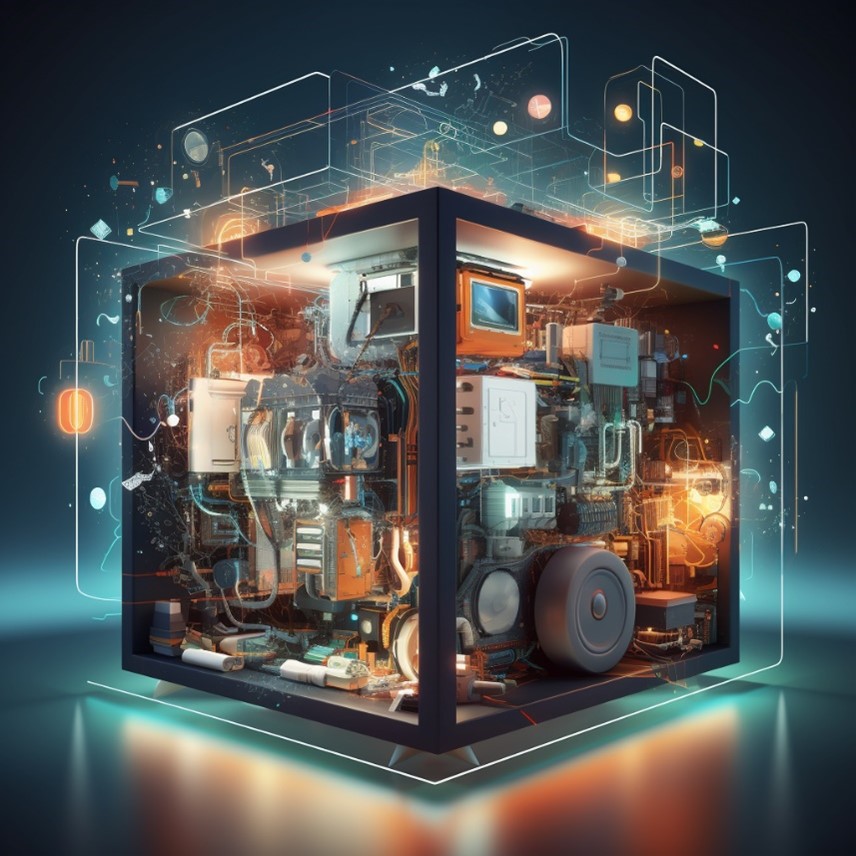
If we fantasize a bit further and consider the AI element represented by the above box as a specialized system for a particular use case in the autonomous driving domain, then we want to understand and learn how we could develop from the construction of this box to a completely different box. This other box could be a distinctly other use-case in the autonomous domain. But it could also stand for a use case in the medical domain, or the energy sector, or in the financial technology domain (see Fig. 03 for other Midjourney dream samples of such AI boxes).
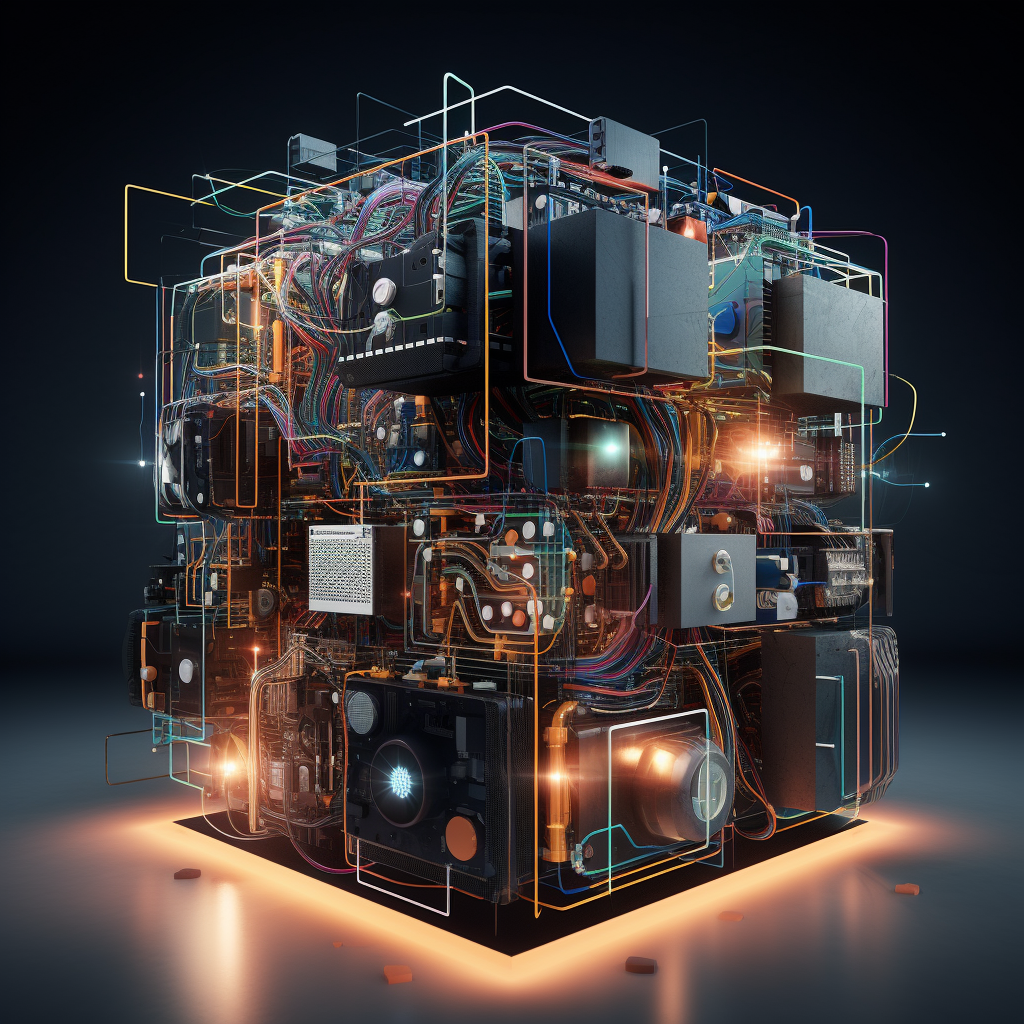
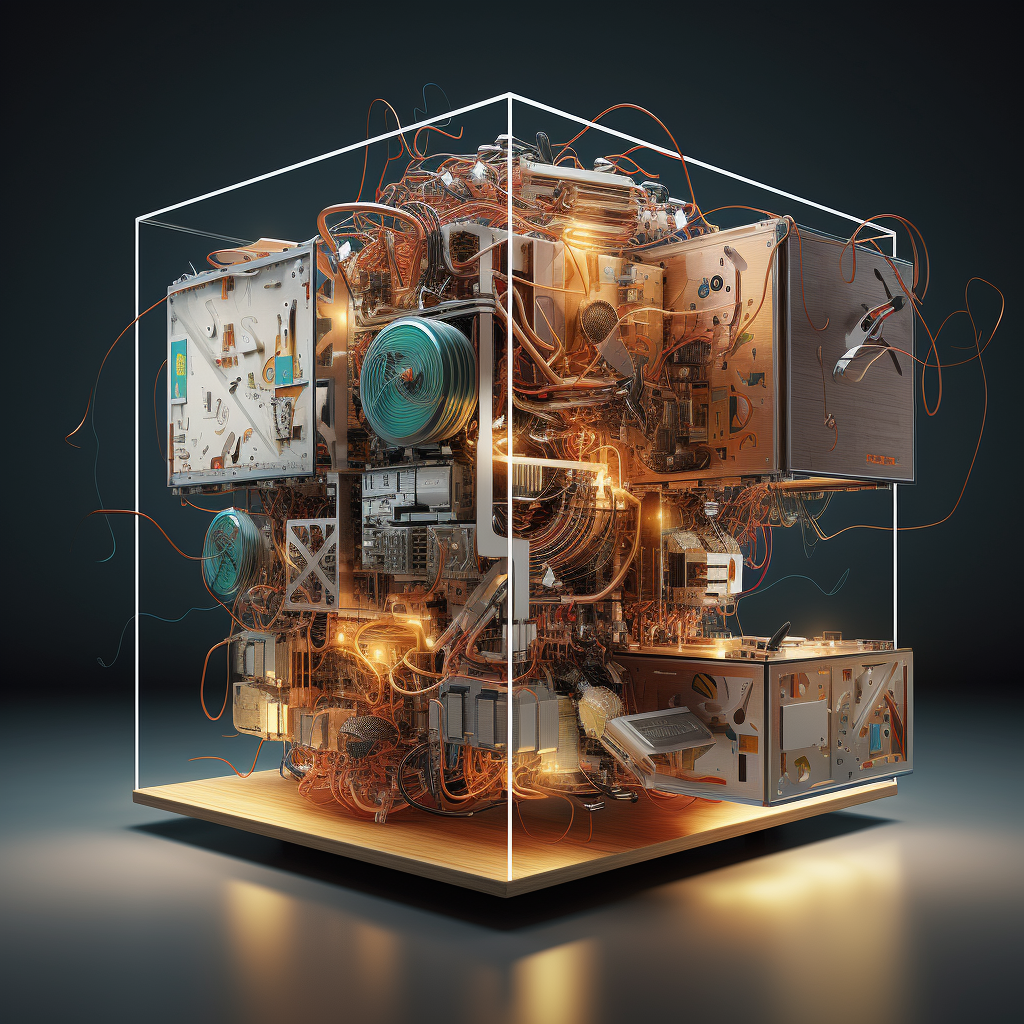
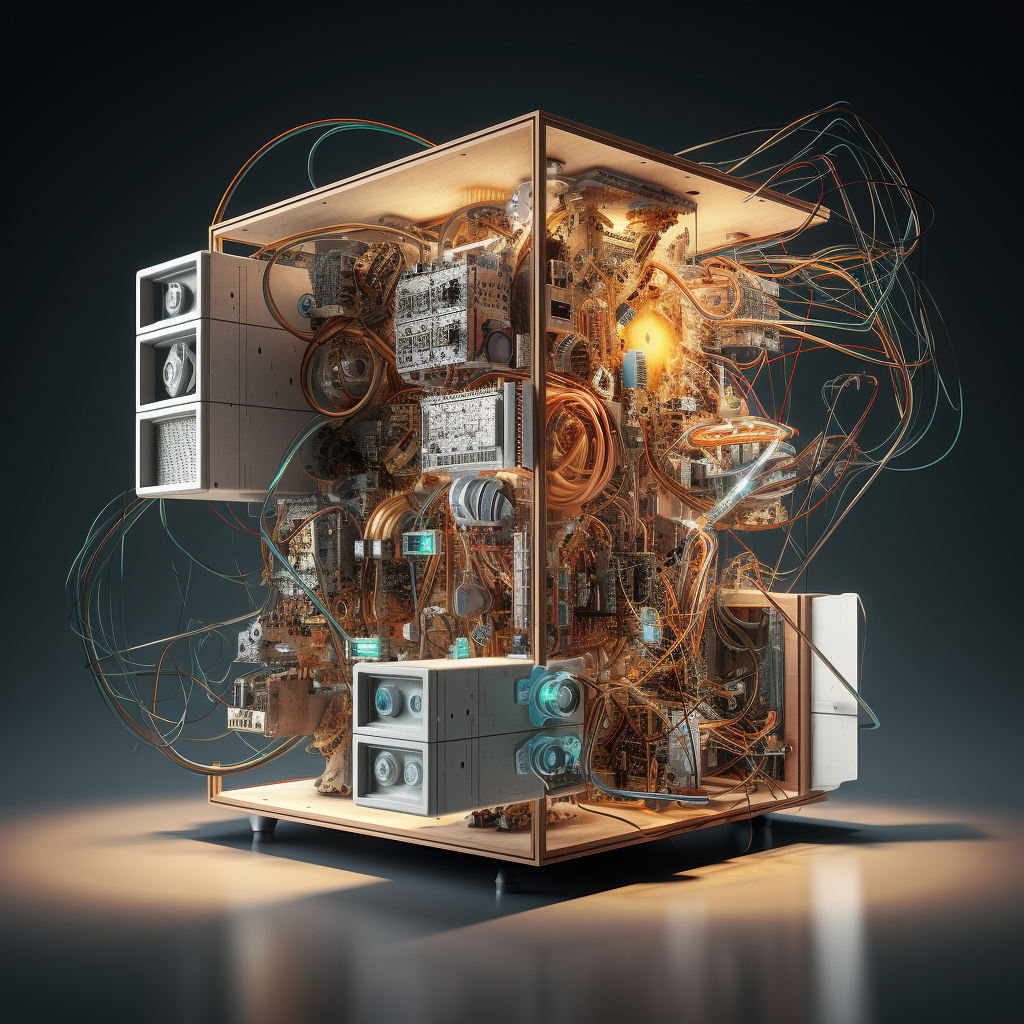
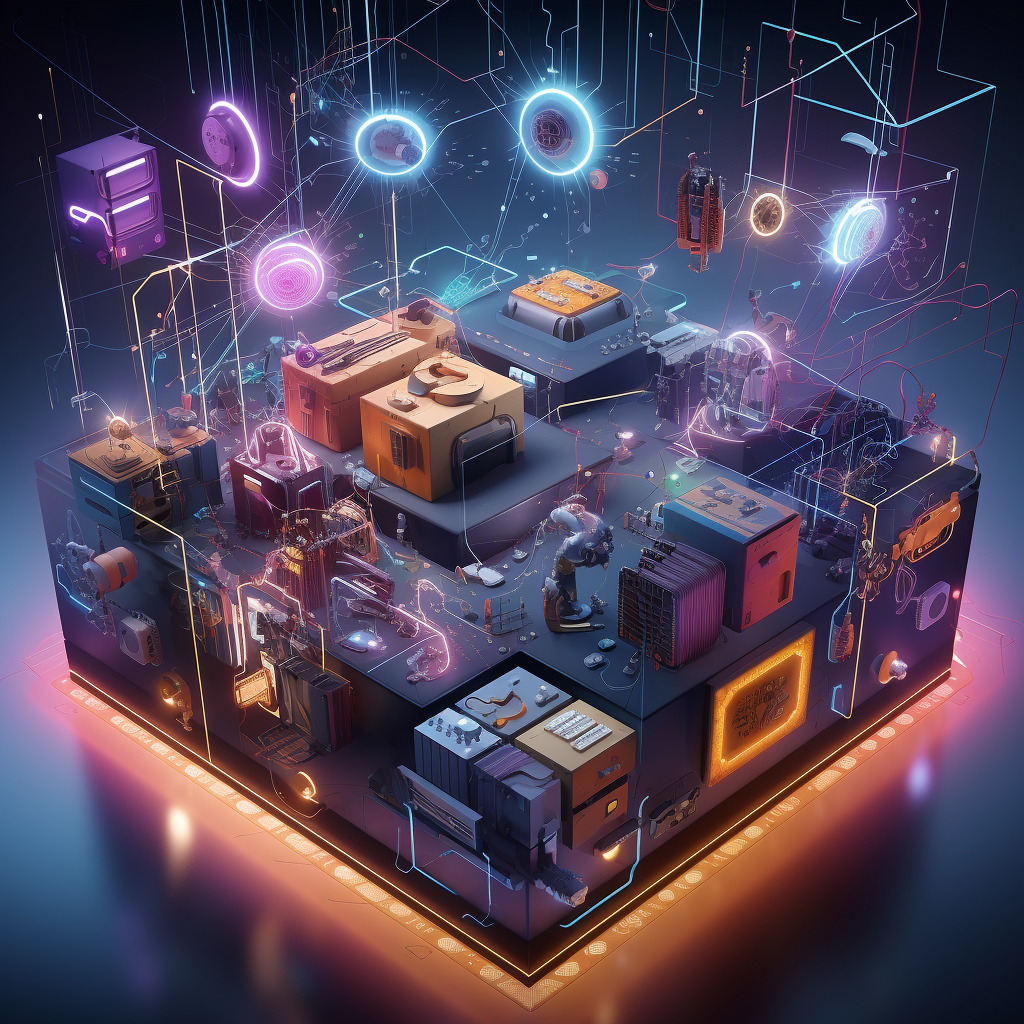
The motivation for me to come up with the concept of such an adaptable AI Assurance system came from my time before I joined diconium. A couple of years ago, I worked as Chief AI Scientist at Lindera GmbH, a small Startup in Berlin. The main product of Lindera which we built back then, is a Software as a Medical Product (SaMD) used for the elderly care sector and for the aim of fall prevention. At the core of the product was an algorithm pipeline based on modern computer vision elements (Convolutional Neuronal Networks). During that time, I learned a whole lot, not only about just the technical parts of building an AI-based medical software product. The most important learning for me from that time was the complexity of ensuring the safety and validity of a medical product with AI/ML elements at its core. This is even more true for a small startup. Back then, a well thought out framework that is automating the development of AI-enabled medical software products from the requirements, over to data processing, to modeling, up to deployment, would have been a dream come true. Hence, when I started my work on the diconium project dealing with safety assurance of AI in automotive domain, the step to the abstraction of the basic concept to any other safety-relevant domain (medical, industrial, financial) was not a long stretch.
We, me and my team, have now reached a first major milestone on our long way to make the vision I had one and a half years ago a reality in the future. On our journey to this bold endeavor, we built an AI system for pneumonia detection based on upper chest x ray images. The aim for it was to achieve a Trusted AI conditional certificate for this blueprint use case. We now proudly can claim that we received this conditional certificate by TÜV Austria Group & SCCH. This was a big effort of the whole team and a big thank you goes out to our starting team with Nicolas Moegelin, Nadja Müller and Prateek Narula. Furthermore, a big thank you goes to David Blumenthal-Barby, Neil Sinclair, Marshall Mykietyshyn and Neginsadat Miriyan for their great work on the development and certification process. Finally, a big thank you goes out also to our directors, Tomas Golabski, Peer Schwirtz, Boris Wolters, Tobias Margarit, and Swantje Kowarsch, for their tremendous amount of support.
This success shall be our first step to help our current and future customers on their way to develop their safety-relevant AI systems. Each of these shall serve us in developing increasingly into the direction of a general Continuous AI Assurance framework. That is my vision for the future.
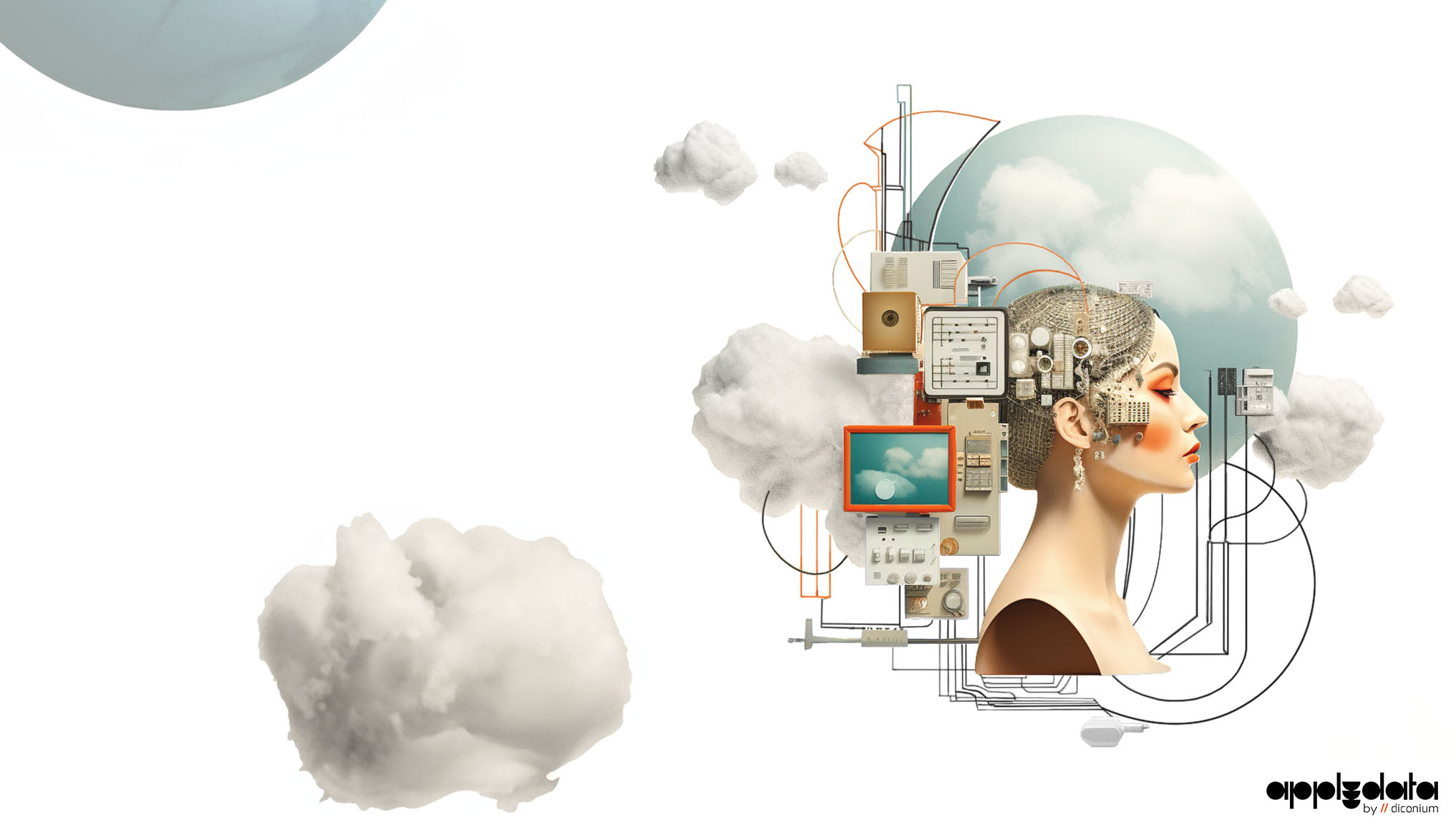