According to a study published by the Fraunhofer-Institute 73% of all data projects fail [1]. One of the main reasons for this is a lack of definition, which describes the connection between the implementation of data-driven solutions and the company’s business strategies. To successfully implement a data project and generate the maximum business value, a solid bridge must be established between the implementation of the data-driven solutions and all crucial business aspects of a company. The Business Analytics Process [2], which is described in more detail below, can be used to create this bridge and to recognize, organize and structure all other important aspects of the data project.
Business Analytics Process
The Business Analytics Process is divided into the four phases: “Framing”, “Allocation”, “Analytics” and “Preparation”. These phases can be run once or several times depending on the requirements [2].
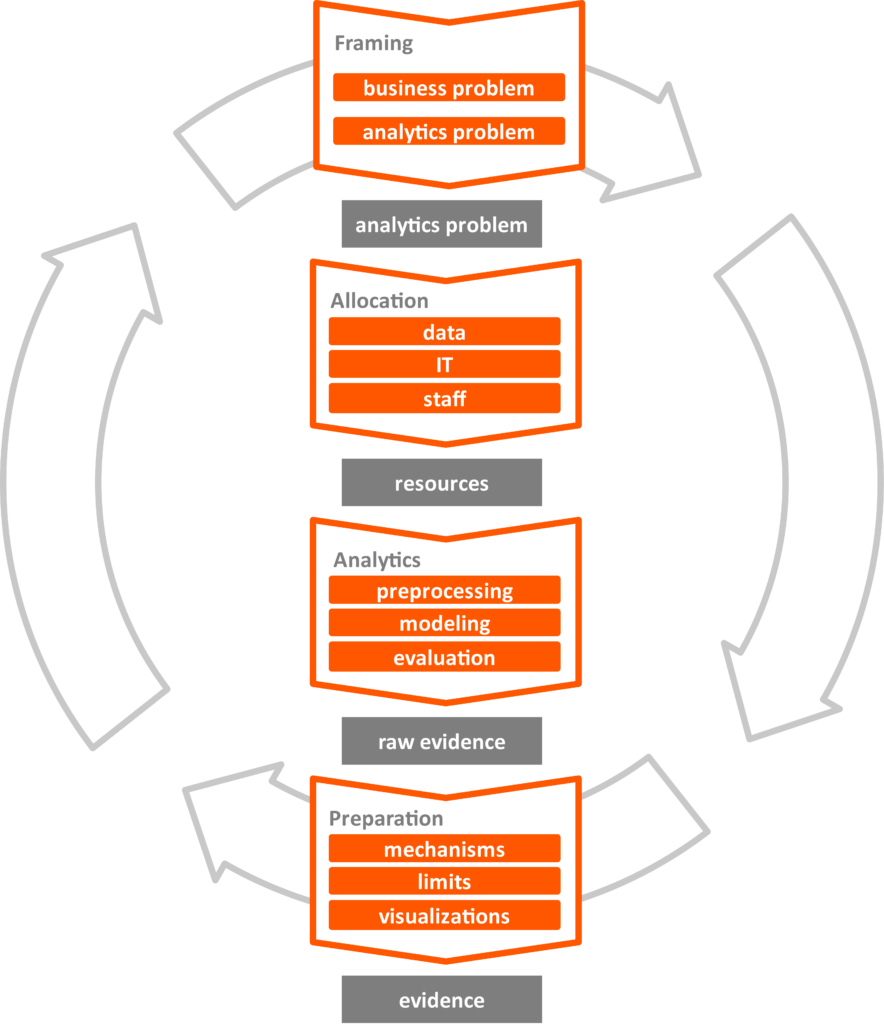
Framing
In the first stage, in the “Framing” phase an economic problem is identified. The identification is usually based on the experiences or observations of managers, tips from external parties or cooperation partners. Next the problem should be operationalized, so that a relevant Business Problem is formed. At the end of the “Framing” phase, an Analytics Problem is defined SMART (Specific, Measurable, Attainable, Relevant, Timed) based on the Business Problem, which maps a solution strategy for the Business Problem and is implemented in further phases.
Allocation
The goal of the “Allocation” phase is to identify and provide the key resources such as data, IT resources, and staff to solve the Analytics Problem defined in the “Framing” phase.
Among other things, the data to be used is defined in this sub-process. The data quality must be ensured as well.
In addition, powerful IT resources such as a cloud environment (e.g. AWS, GCP, Microsoft Azure) are provided, which have sufficient capacity to solve the Analysis Problem.
According to the needed skillset, the team is constructed by dependencies on the Analytics Problem: data acquisition and storage, data preparation, data analysis, visualization, deployment, security and legaling.
Analytics
In the “Analytics” phase a data-driven extraction of raw evidence is carried out in order to solve the Analytics Problem defined in the “Framing” phase. Here the data from the “Allocation” phase is prepared and provided. Next a data analysis is performed. This can be for example a descriptive analysis, a predictive analysis or a prescriptive analysis.
Finally, the results of the “Analytics” phase are evaluated. For example: the used machine learning algorithms are compared with each other, and the output data of the algorithms are evaluated.
Preparation
In the final “Preparation” phase of the Business Analytics Process the raw evidence from the “Analytics” phase is prepared and visualized, whereby the final evidence is resulted. In addition, mechanisms of the final evidence are explained and, recommendations for action should be derived. This final evidence can be optimally used by users in a company. Validity limits of the final evidence are also established in this sub-process. For explanation: if the gained evidence is based on certain customer behavior and this customer behavior will change in the future, then this evidence is no longer valid.
Apply Data for Business Value
We believe at diconium that there is huge potential in the data of every company and that with the Business Analytics Process a large business value can be achieved for the company.
The Business Analytics Process can be used flexibly in various data-driven projects. For example, the Business Analytics Process can support the reduction of order returns in retail by creating a customized data-driven solution with various data (such as sales data, warehouse data or social media data) and company strategies.
The major strength of the Business Analytics Process is to create a robust bridge between the implementation of a data-driven solution and important business requirements of a company. This ensures that the results of a data project will contribute to the success of a company.
Sources: [1] https://publica-rest.fraunhofer.de/server/api/core/bitstreams/9ed9840e-2f1a-4e0e-8e12-74ae965f1bd0/content [2] Mischa Seiter, „Business Analytics: Wie Sie Daten für die Steuerung von Unternehmen nutzen“, 2. Auflage, 2019, Vahlen